GLAI’s innovative retraining approach
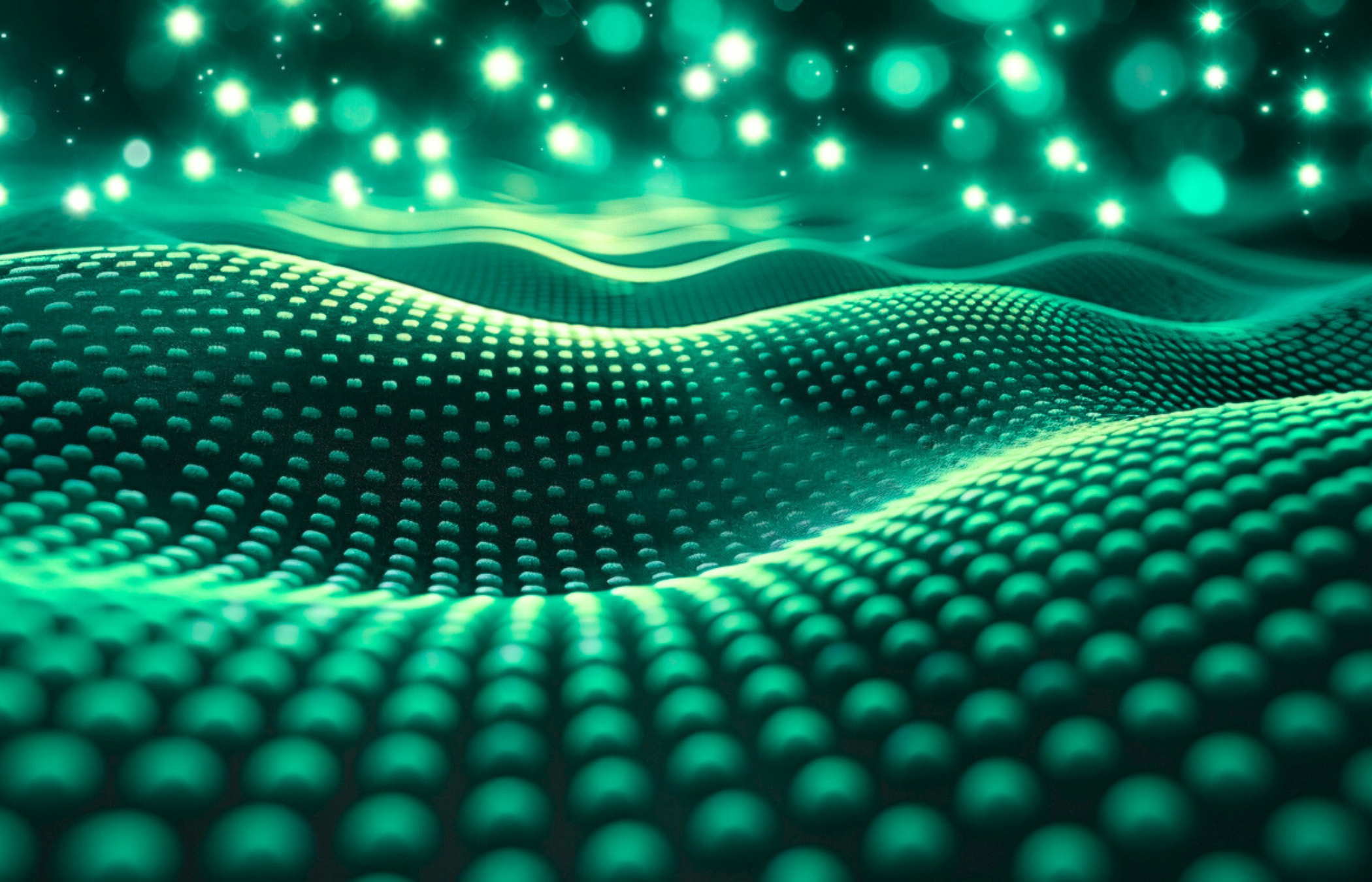
December 16, 2024
By Qsimov
December 16, 2024
By Qsimov
Artificial intelligence has become a cornerstone of technological innovation, driving advancements across industries. As its adoption grows, the demand for more efficient, adaptable, and sustainable AI solutions becomes increasingly critical. Retraining AI models efficiently is one of the key challenges organizations face.
Continual learning stands as a pivotal element of intelligence, embodying the capacity to build upon existing knowledge by progressively learning from new experiences. This ability is crucial for any entity, be it biological or artificial, navigating an ever-changing environment. Humans excel at this, as our brains can seamlessly acquire new skills without erasing or diminishing those previously mastered. This natural aptitude for incremental learning serves as an inspiration for designing artificial systems capable of adapting and thriving in dynamic contexts.
Deep neural networks have revolutionized artificial intelligence with their remarkable representation learning capabilities. However, their inability to learn continuously presents significant challenges, especially as they require extensive training on large datasets to maintain high performance.
This limitation is particularly critical in digital twin applications, where continual learning is essential to minimize reliance on pre-deployed solutions. As a result, continual learning has emerged as a pivotal area of research in deep learning, representing one of the field's most pressing challenges and opportunities for innovation.
AI models learn from data, and their accuracy depends on the quality and recency of the data they process. However, as data changes, whether due to evolving consumer behaviors, emerging trends, or shifts in environmental conditions, models must be retrained to stay relevant.
Consider these scenarios:
Retraining enables AI models to incorporate such new information. But traditional retraining methods face three primary challenges:
Inefficiency: Retraining often requires reprocessing vast datasets, leading to increased computational costs and extended processing times.
Catastrophic forgetting: When models learn new data, they may overwrite or lose previous knowledge, compromising their overall accuracy.
Energy consumption: Prolonged retraining processes consume significant energy, increasing costs and leaving a heavier environmental footprint.
GLAI introduces a paradigm shift by addressing these challenges head-on with groundbreaking innovations. Its capabilities redefine how retraining is approached, enabling businesses to keep their AI models agile, accurate, and efficient.
Traditional retraining methods often require the AI model to revisit its entire dataset, even when only a small subset of new data needs to be integrated. This not only wastes time but also consumes unnecessary resources.
GLAI’s revolutionary approach to retraining is rooted in a system design that separates structural and quantitative knowledge, enabling unparalleled efficiency. Unlike traditional deep neural networks (DNNs), which often require processing vast amounts of old and new data during retraining, GLAI processes only the new samples. This is achieved through an innovative linear model that emulates the behavior of DNNs by subsetting the model for each specific sample.
The linear model retains the quantitative knowledge, while the structural knowledge governs the subsetting process. This design not only accelerates retraining times but also ensures that knowledge from prior data is preserved, offering a streamlined and effective solution for AI systems requiring frequent updates.
This approach:
In an era where sustainability is a global priority, GLAI offers a solution that aligns with environmentally conscious AI practices. By dramatically reducing retraining times, GLAI:
GLAI goes beyond optimizing retraining processes; it redefines the possibilities of AI system design and deployment. With seamless integration capabilities, GLAI effortlessly adapts to existing workflows, enabling businesses to harness its advanced features without the need for extensive infrastructure changes.
Whether operating in centralized servers or edge environments, GLAI meets diverse operational requirements with ease. Its performance is equally impressive, achieving inference accuracy that rivals, or even surpasses, traditional neural networks, demonstrating that speed and efficiency can coexist with high-quality outcomes.
Moreover, GLAI prioritizes explainability and transparency, transforming AI models from opaque systems into tools that provide actionable insights. By revealing how specific inputs influence outputs, GLAI empowers users to refine and improve models with confidence. This transparency is particularly critical in high-stakes sectors like healthcare or finance, where understanding the rationale behind AI decisions is essential for building trust and ensuring compliance.
In healthcare, GLAI enables AI systems to adapt seamlessly to new patient data through incremental retraining, ensuring improved diagnostic accuracy while preserving insights from historical records.
In the finance sector, fraud detection models can undergo rapid retraining to respond to emerging threats in real time, safeguarding assets with unmatched efficiency. In retail, recommendation engines benefit from retraining that incorporates seasonal trends, keeping suggestions relevant and dynamic without discarding valuable prior data.
Meanwhile, in the realm of autonomous systems, such as self-driving cars and drones, GLAI's ability to retrain on new scenarios ensures continuous learning and adaptation while upholding rigorous safety standards. GLAI proves that incremental retraining is not just a technical advancement, it is a cornerstone of innovation across industries.
GLAI isn’t just a tool, it’s a transformative approach to AI. By redefining retraining, it enables businesses to stay ahead in competitive markets while championing sustainable innovation. Stay connected with Qsimov as we continue to push the boundaries of what’s possible in AI and quantum computing. Together, let’s shape a smarter, greener, and more efficient technological future.